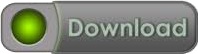
The interpretation of the intercept parameter, b, is, "The estimated value of Y when X equals 0." The linear regression interpretation of the slope coefficient, m, is, "The estimated change in Y for a 1-unit increase of X." X is simply a variable used to make that prediction (eq.

Keep in mind that Y is your dependent variable: the one you're ultimately interested in predicting (eg. The calculator above will graph and output a simple linear regression model for you, along with testing the relationship and the model equation. Linear regression calculators determine the line-of-best-fit by minimizing the sum of squared error terms (the squared difference between the data points and the line).
Linear equation calculator how to#
While it is possible to calculate linear regression by hand, it involves a lot of sums and squares, not to mention sums of squares! So if you're asking how to find linear regression coefficients or how to find the least squares regression line, the best answer is to use software that does it for you. Variables (not components) are used for estimation Have a look at our analysis checklist for more information on each:

If you're thinking simple linear regression may be appropriate for your project, first make sure it meets the assumptions of linear regression listed below. The formula for simple linear regression is Y = mX + b, where Y is the response (dependent) variable, X is the predictor (independent) variable, m is the estimated slope, and b is the estimated intercept. The quantity y i − x i T b, called the residual for the i-th observation, measures the vertical distance between the data point ( x i, y i) and the hyperplane y = x T b, and thus assesses the degree of fit between the actual data and the model.Linear regression is one of the most popular modeling techniques because, in addition to explaining the relationship between variables (like correlation), it also gives an equation that can be used to predict the value of a response variable based on a value of the predictor variable. Suppose b is a "candidate" value for the parameter vector β. Suppose the data consists of n Estimation
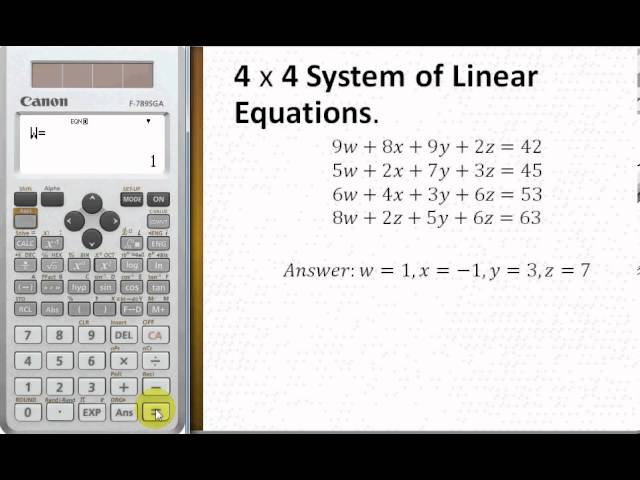
Here the ordinary least squares method is used to construct the regression line describing this law. Okun's law in macroeconomics states that in an economy the GDP growth should depend linearly on the changes in the unemployment rate. Under the additional assumption that the errors are normally distributed with zero mean, OLS is the maximum likelihood estimator that outperforms any non-linear unbiased estimator. Under these conditions, the method of OLS provides minimum-variance mean-unbiased estimation when the errors have finite variances. The OLS estimator is consistent for the level-one fixed effects when the regressors are exogenous and forms perfect colinearity (rank condition), consistent for the variance estimate of the residuals when regressors have finite fourth moments and-by the Gauss–Markov theorem- optimal in the class of linear unbiased estimators when the errors are homoscedastic and serially uncorrelated. The resulting estimator can be expressed by a simple formula, especially in the case of a simple linear regression, in which there is a single regressor on the right side of the regression equation. Geometrically, this is seen as the sum of the squared distances, parallel to the axis of the dependent variable, between each data point in the set and the corresponding point on the regression surface-the smaller the differences, the better the model fits the data.
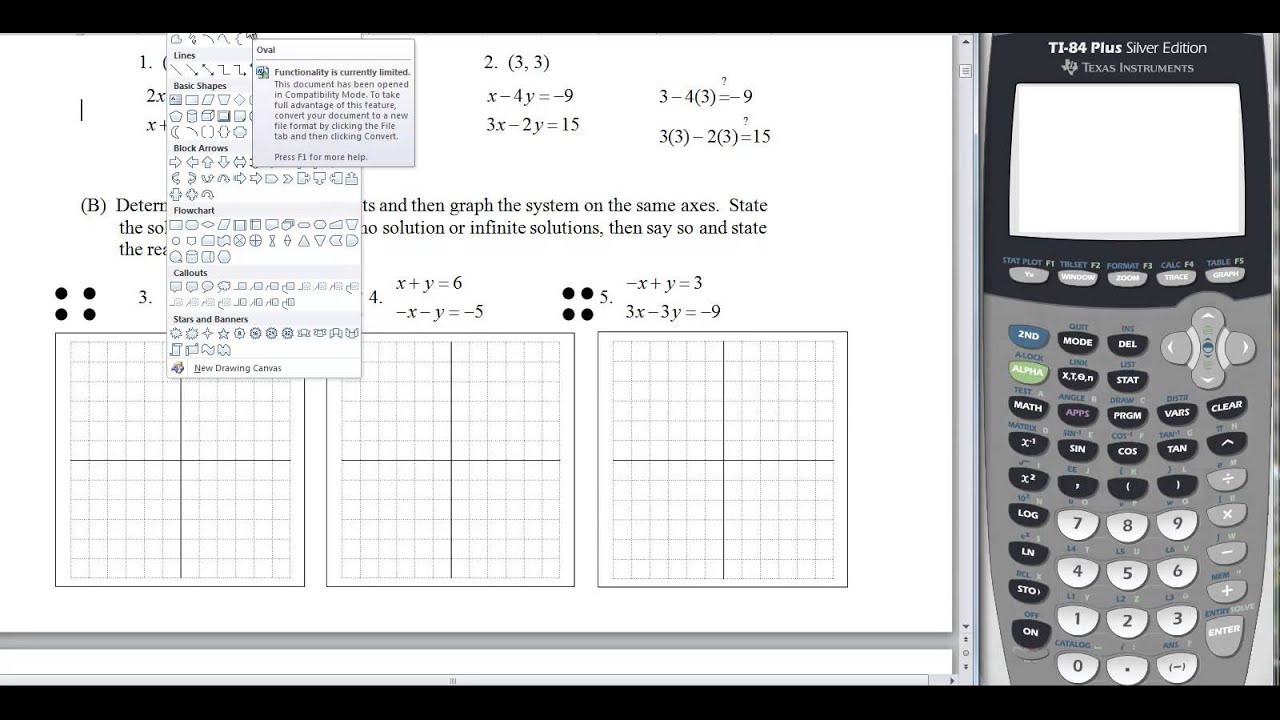
In statistics, ordinary least squares ( OLS) is a type of linear least squares method for choosing the unknown parameters in a linear regression model (with fixed level-one effects of a linear function of a set of explanatory variables) by the principle of least squares: minimizing the sum of the squares of the differences between the observed dependent variable (values of the variable being observed) in the input dataset and the output of the (linear) function of the independent variable.
Linear equation calculator series#
Method for estimating the unknown parameters in a linear regression model Part of a series on
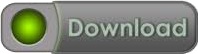